Case Study
Personalized voucher recommendations for a bank’s customers
Developing a rule-based recommendation engine to individualize offers based on a customer’s past transactions.
Our client asked us to increase the relevance and conversion rates of voucher offerings presented in their app (for their own product and their loyalty network of retail partners).
Prior to the project, the bank’s product recommendations were based on simple rules and randomization services. As the conversion rates were relatively low, management wanted to improve the opportunities of their own and their retail partner’s loyalty program. This meant a complete redesign of their app voucher structure through a personalized voucher system.
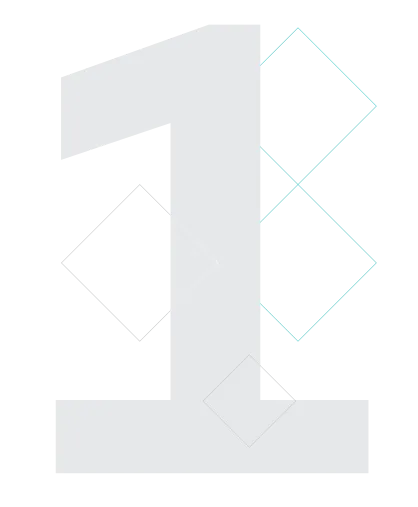
We made use of previously untapped proprietary data to substantially increase the relevance of their loyalty program.
In this stage, we set up a new system for recommendations based on customer and transaction data as well as look-alike audiences.
We followed a scientific approach to segment the bank’s customers. This was achieved by:
- Setting up a rule-based product prediction model based on customers’ and a look-alike audience’s spending behavior
- Testing of generated recommendations in interviews with selected customers to confirm our recommendations and getting insights on their preferred design
- Adjusting the look of the voucher system based on the customers’ usability experiences
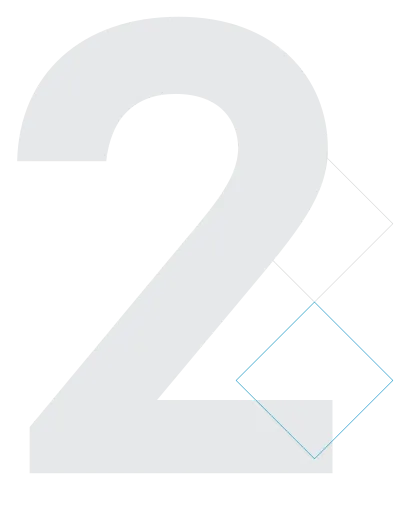
78 percent of the bank’s customers preferred the optimized recommendations.
We have introduced personalized voucher recommendations that substantially increased conversion rates on the bank’s loyalty network app.
We could bring the bank’s user experience to a new level by customizing the app with:
- Segmentation for each user based on customer data (age, gender, income, …) and past purchasing behavior
- Multiple sets of rules based on the past transactions of the individual customer and their look-alike audience as well as individual information (children, pet, car, …)
- Integration of the customer’s past interactions with the loyalty partners correlated with their spending
In the last step, we even prepared an A/B test to allow our client to further analyze and improve our recommendations after the launch of the app to verify the effectiveness of the new algorithm.
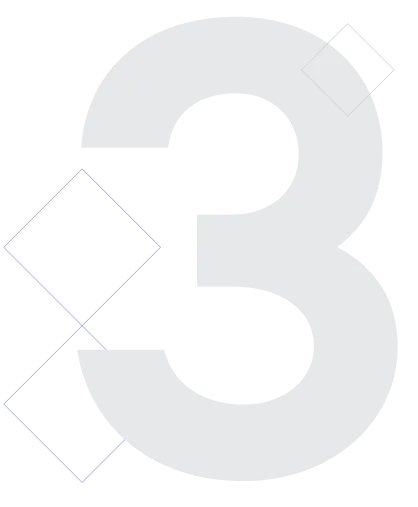
Contact us
Our experts are always happy to discuss your issue. Reach out, and we’ll connect you with a member of our team.