Is your pricing system up to date? In our latest article, our banking expert discusses the crucial importance of price updates for credit products.
After the second quarter of 2023, the number of business bankruptcies in the EU has climbed to the highest level since Eurostat’s first data collection in 2015. The end of pandemic support measures and inflationary cost increases left their scars on most profit and loss statements.
To be more specific, financing costs increased massively. Due to these changing market conditions, companies with a low interest coverage ratio (ratio of EBIT to interest expenses) will be particularly affected. Consumers are also witnessing a similar trend. In Germany, for example, personal bankruptcies almost doubled in 2021 compared to the year before.
The impact of the lower-for-longer interest rate environment is evident in its turnaround. For lending businesses, these developments are also worrying for another reason. Many pricing systems work with standardized default risk cost components that are literally out of joint. The standardized risk costs (STRC) used in these systems are usually based on historical data points from the last five to ten years. In times of increasing financial volatility, the forecasting quality of these systems drops significantly. As these systems are very often directly linked with pricing, their predictive performance should be critically reviewed.
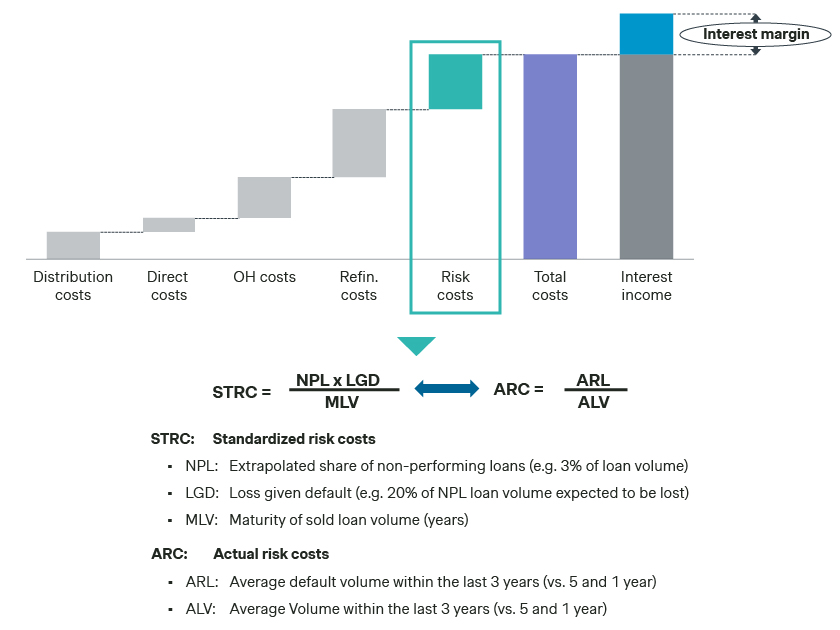
As shown in Figure 1, one way to assess the validity of the predicted risk costs is to compare the actual risk costs (ARC) with the assumed STRC. If the predicted risk costs deviate significantly from the ARC, it’s strongly recommended to update both the STRC and the entire pricing model. However, an update should also be considered when a shrinking market share is observed. In our experience, next to sales-related reasons, price-related factors (pricing system not sufficiently granular, interest margins not differentiated, price levels not competitive, etc.) are frequently the primary cause of market share decline.
Price updates in practice
Based on a few cases from our consulting practice, we would like to illustrate the range of price update possibilities:
- Bank A, providing consumer loans, has a decentralized pricing system. Local controlling units calculate STRCs, which are then used to determine prices. An analysis shows that the STRCs are clearly too low. One reason for this is that the local units are averaging secured with unsecured loans when calculating STRC.
- Bank B, providing business loans, has a centralized pricing system. To offer customers an immediate indicative rate, a new offering process has been set up and a new scoring procedure was developed. However, the calculation is still based on an Excel sheet that is linked to numerous old data points in various files.
- Bank C has developed a dynamic pricing system that utilizes machine learning as a challenger to the existing pricing system. It continuously optimizes and updates itself. The system works with various algorithms to determine the optimum interest rate. Number and connection between the variables go far beyond usual pricing systems. In tests, however, this pricing system does not perform significantly better across all segments. Additionally, pricing became largely a “black box”, as it is not transparent how prices are derived, and which variables exert the strongest impact.
Optimization approaches
These different approaches demonstrate the extent to which a pricing system should be updated depends mainly on the structural situation. If, for example, the comparison of STRC and ARC shows significant deviations, this can be the starting point for investigation (see Figure 1). However, we recommend looking at the pricing system holistically and updating it structurally. We often find that important price parameters are already in place in the operating software but are overlooked by the pricing system.
Regardless of whether the goal is a fundamental structural revision or just a price update of the existing interest rate matrix, our experience has shown that a systematic approach to optimization is the right way. The recommended steps are shown in Figure 2 and comprise analysis, design, and simulation before deciding on the new pricing system or price updates.
Transparency phase | Concept phase | Simulation phase | Decision |
---|---|---|---|
Analysis of the current pricing system
| Conception/update of new pricing system
| Fine-tuning new pricing system / price update
| Decision for new pricing system/price update
|
Analysis of commission/bonification model, if applicable | Concept/update product design | Finalization of product design or update | Decision for new product design / updates |
Analysis of product design | Integration of sales forces' view | Installation test & learn environment for further optimization |
Here we outline our experience with the individual phases.
- Transparency phase: Next to analyzing the individual contract portfolio, it is particularly important to look at the customer level, as key data points (e.g., creditworthiness ratings, exposure volumes, maximum loan amounts) are located at this level. Depending on the pricing logic (negotiated vs. predetermined prices), the sales commission model should also be included in the analyses. During this phase, it is crucial to look at the interaction of the different pricing components and to review their logic and level. The product design should also be considered in this context. For example, bundling various features at product level can result in a more favorable price (“deal effect”).
- Concept phase: Once the transparency is established, optimization can begin. This includes both price differentiation (e.g., creditworthiness rating/risk, term, volume, interest rates of existing contracts) and price logic (interaction of price parameters, smoothing). The amendment of pricing systems usually requires changes to the operating software. If the price points are parameterized and the goal is to only update the price levels, the required effort should be minimal. For concepts with major changes, the necessary time frame for implementation should be clarified with the IT department as early as possible. Depending on the situation, the sales department should also be involved in this phase.
- Simulation phase: There are different price elasticities within the credit decision process. The first and foremost question is: “Will the loan application be decided positively?”. Thereafter, the acceptance elasticity comes into play: “Will the offered conditions be accepted?”. A simulation of the new pricing model or the new price levels should be carried out for iterative optimization purposes and to estimate the effects. The perceived price positions of the competition should also be included within the simulation so that a forecast of the results can be produced. The simulation can provide evidence that demonstrates an update is the better alternative compared to the status quo.
Next generation: AI-powered pricing systems
The next generation of AI-supported pricing systems has the potential to automate the phases we have described above. The requirement for them to run autonomously would be constant self-optimization without external guidance or corrections. To achieve this, various modules would need to be connected, continuously integrating extensive internal data sources and current market and competitor data points. Decision optimization, simulation, and visualization or reporting could be almost completely taken over by AI.
An interesting point to consider is whether the AI should have a role in deciding new price levels. Such a “handover” could only take place if the AI pricing system produces significantly better results in any situation. A proof of this is difficult to imagine since environmental variables are constantly changing. It is hard to envision a proof for this due to the constant fluctuation of environmental factors. For example, if the AI was mainly trained with data from the low-interest phase, it cannot be assumed that the adjustments made by the AI under the current highly volatile conditions are optimal. Additionally, the question remains whether the responsible management would be willing to delegate such responsibilities.
For these reasons, the current trend is to frame AI more as an intelligent assistance system that excels in improving tasks such as data integration (updating and integrating extensive external and internal data volumes), scenario evaluation, and visualization. In this respect, it can be assumed that the AI component can make a significant contribution to more granular, up-to-date, and improved pricing. The current practice of updating pricing systems could then be observed in the rear mirror of an AI assistant.
Interested to know more? Reach out to us today.